Table Of Content

Each measurement or observation is made on an item denoted as an experimental unit. Again, because neither independent variable in this example was manipulated, it is a non-experimental study rather than an experimental design. This is important because, as always, one must be cautious about inferring causality from non-experimental studies because of the threats of potential confounding variables.
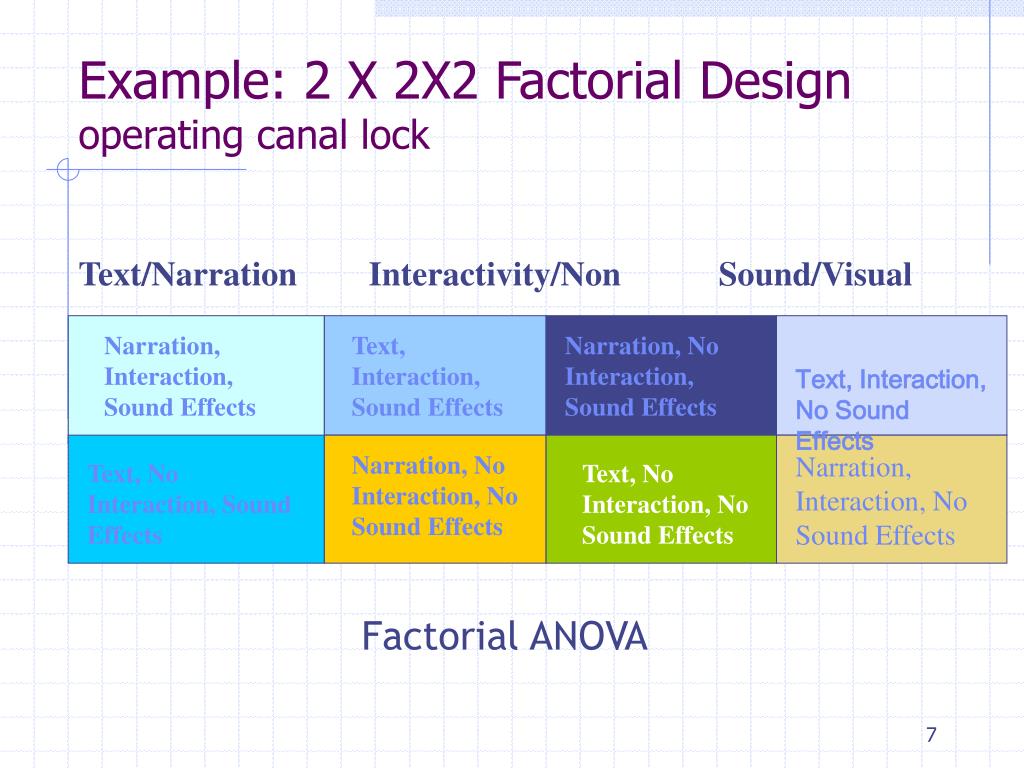
Factorial experiment
Some examples of bar and line graphs are presented in the margin, and two example tables are presented below. As expected, we the average height is 6 inches taller when the subjects wear a hat vs. do not wear a hat. So, the main effect of wearing hats is to add 1 inch to a person’s height. As expected, we see that the average height is 1 inch taller when subjects wear shoes vs. do not wear shoes. So, the main effect of wearing shoes is to add 1 inch to a person’s height. Introduction to Statistics is our premier online video course that teaches you all of the topics covered in introductory statistics.
IX. Chapter 9: Factorial Designs
If we made a separate graph for the main effect of shoes we should see a difference of 1 inch between conditions. Similarly, if we made a separate graph for the main effect of hats then we should see a difference of 6 between conditions. However, we can also perform a two-way ANOVA to formally test whether or not the independent variables have a statistically significant relationship with the dependent variable. Plotting the means is a visualize way to inspect the effects that the independent variables have on the dependent variable.
Visualizing Main Effects & Interaction Effects
Since factorial designs have more than one independent variable, it is also possible to manipulate one independent variable between subjects and another within subjects. For example, a researcher might choose to treat cell phone use as a within-subjects factor by testing the same participants both while using a cell phone and while not using a cell phone. But they might choose to treat time of day as a between-subjects factor by testing each participant either during the day or during the night (perhaps because this only requires them to come in for testing once).
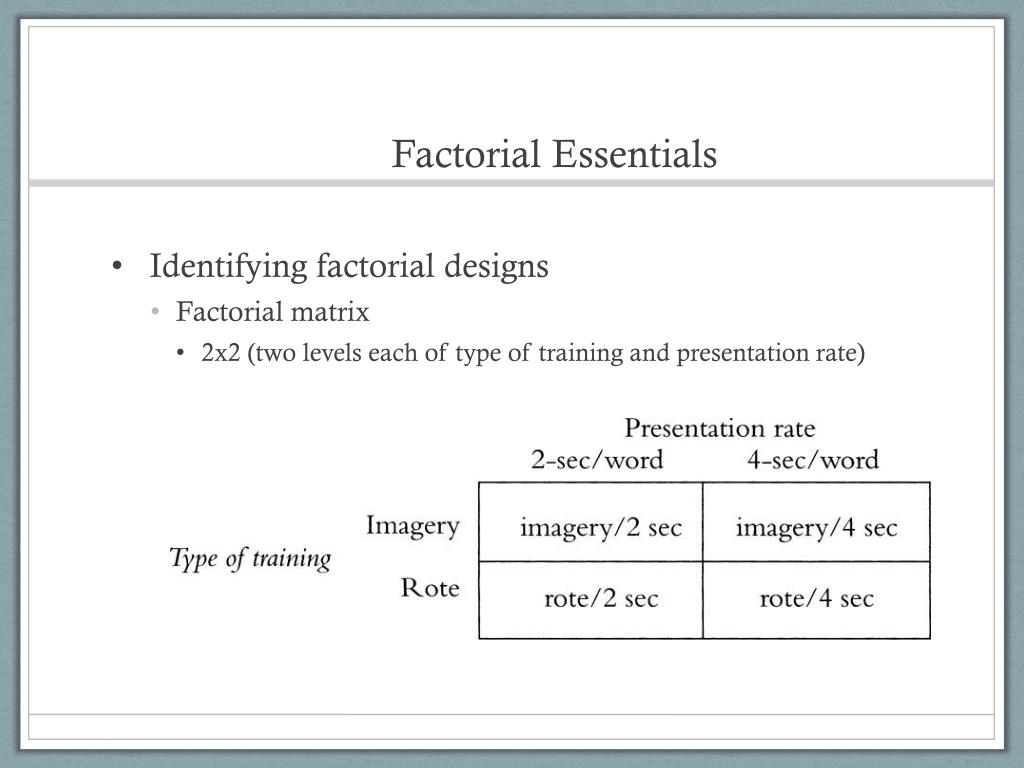
Book traversal links for Lesson 5: Introduction to Factorial Designs
It is tempting to take advantage of the efficiency of the factorial experiment and use it to evaluate many components since power is unrelated to the number of factors, and therefore, a single experiment can be used to screen many components. However, the number of factors used and the types and number of levels per factor can certainly affect staff burden. A 5-factor design with 2-levels/factor yields some 32 unique combinations of components (Table 1), and requires that at least five different active or “on” ICs be delivered. Moreover, if instead of “off” or no-treatment conditions, less intensive levels of components are used, then even more ICs must be delivered (albeit some of reduced intensity). In general, if the major goal of a study is to contrast directly one “treatment” with another treatment (e.g., a control treatment), then an RCT is usually the best choice. Note that here “treatment” is used to connote a set of intervention components (ICs); e.g., a particular type, dose, and duration of medication, type of counseling that is delivered for a particular number of sessions of a particular duration, and so on.
Factorial Experimental Design
In looking at the interactions, AB, is not significant, BC is not significant, and the ABC are not significant. To restate this, in terms of A, the A effect is the difference between the means at the high levels of A versus the low levels of A, whereas the coefficient, \(\alpha_i\), in the model is the difference between the marginal mean and the overall mean. So the Yates "effect" is twice the size of the estimated coefficient αi in the model, which is also usually called the effect of factor A.
Again, because neither independent variable in this example was manipulated, it is a non-experimental study rather than an experiment. If an investigator anticipates severe problems from including a particular factor in an experiment, perhaps due to its nature or the burden entailed, s/he should certainly consider dropping it as an experimental factor. Indeed, the MOST approach to the use of factorial designs holds that such designs be used to decompose a set of compatible ICs, ones that might all fit well in an integrated treatment package (to identify those that are most promising). That is, one should include only those ICs that are thought to be compatible, not competitive. As a further example, the effects of three input variables can be evaluated in eight experimental conditions shown as the corners of a cube.
2.3. Assigning Participants to Conditions¶
How design of experiments lowers costs in R&D - Scientific Computing World
How design of experiments lowers costs in R&D.
Posted: Wed, 29 Mar 2023 11:18:22 GMT [source]
Once all desired changes have been made, click "OK" to perform the analysis. All of the plots will pop-up on the screen and a text file of the results will be generated in the session file. The names of each response can be changed by clicking on the column name and entering the desired name. In the figure, the area selected in black is where the responses will be inputted. For instance, if the purity, yield, and residual amount of catalyst was measured in the DOE study, the values of these for each trial would be entered in the columns. Minitab 15 Statistical Software is a powerful statistics program capable of performing regressions, ANOVA, control charts, DOE, and much more.
Specifically, they indicate how much the dependent variable changes for each one-unit change in the independent variable. The effect of one independent variable can depend on the level of the other in several different ways. The combined main effects are significant as seen in the combined summary table. And the individual terms, B, C, D, BC and BD, are all significant, just as shown on the normal probability plot above. Notice also the use of the Yates notation here that labels the treatment combinations where the high level for each factor is involved. If only A is high then that combination is labeled with the small letter a.
In short, maintaining treatment delivery fidelity may take more care, training and supervision in a factorial experiment than in an RCT. Thus, investigators must decide if they wish to directly compare two treatment conditions (and these may be multicomponential) with one another, without the results being affected by the presence of other experimental factors being manipulated. Some experiments involve the study of the effects of multiple factors. A full factorial design, also known as fully crossed design, refers to an experimental design that consists of two or more factors, with each factor having multiple discrete possible values or “levels”. This experimental design method involves manipulating multiple independent variables simultaneously to investigate their combined effects on the dependent variable. When researchers study relationships among a large number of conceptually similar variables, they often use a complex statistical technique called factor analysis.
Minitab is especially useful for creating and analyzing the results for DOE studies. It is possible to create factorial, response surface, mixture, and taguchi method DOEs in Minitab. Ignoring the first row, look in the last stage and find the variable that has the largest relative number, then that row indicates the MAIN TOTAL EFFECT. The Main Total Effect can be related to input variables by moving along the row and looking at the first column. If the row in the first column is a2b1c1 then the main total effect is A.
All rights are reserved, including those for text and data mining, AI training, and similar technologies. For all open access content, the Creative Commons licensing terms apply. Additional modifications to the design include randomizing and renumbering the design. These are very straightforward modifications which affect the ordering of the trials.
These plots are different ways to present the statistical results of the analysis. Examples of these plots can be found in the Minitab Example for Centrifugal Contactor Analysis. The alpha value, which determines the limit of statistical significance, can be chosen in this menu also. A common one to select is "Residuals versus fits" which shows how the variance between the predicted values from the model and the actual values. The following Yates algorithm table was constructed using the data from the interaction effects section.
I’m passionate about statistics, machine learning, and data visualization and I created Statology to be a resource for both students and teachers alike. My goal with this site is to help you learn statistics through using simple terms, plenty of real-world examples, and helpful illustrations. The point of this example is that although the B factor is not significant as it relates to the response, percentage of product defects - however if you are looking for a recommended setting for B you should use the low level for B.
Time series analysis is used to analyze data collected over time in order to identify trends, patterns, or changes in the data. Inferential statistics are used to make inferences or generalizations about a larger population based on the data collected in the study. This design involves grouping participants within larger units, such as schools or households, and then randomly assigning these units to different treatment groups. A traditional experiment would involve randomly selecting different tanks of fish and feeding them varying levels of the additive contained within the feed, for example none or 10%. Another important use of complex correlational research is to explore possible causal relationships among variables.
A null outcome situation is when the outcome of your experiment is the same regardless of how the levels within your experiment were combined. From the example above, a null outcome would exist if you received the same percentage of seizures occurring in patients with varying dose and age. The graphs below illustrate no change in the percentage of seizures for all factors, so you can conclude that the chance of suffering from a seizure is not affected by the dosage of the drug or the age of the patient.
No comments:
Post a Comment